Simplified and efficient upgrade
Elevate your trading game with our state-of-the-art Artificial Intelligence upgrades. Almost all indicator-based trading systems are eligible for transformation into smart, AI-driven systems, preserving the original trading logic for seamless integration. Our team of experts will work with you to ensure a smooth transition and the best possible outcome. Get ready to see improved accuracy, faster decision-making, and boosted efficiency in your trading strategy.
OPTIMAL Portfolio management
Achieve superior portfolio performance with our AI-powered management solutions. Our technology automatically optimizes asset allocation and minimizes system risks based on real-time market analysis. Say goodbye to manual, time-consuming portfolio management and experience the power of AI-driven optimization. Let us help you reach your investment goals with confidence and ease
Standard AI Project Temaplate
Streamline your AI projects with our standard project templates. Built on a foundation of mathematical accuracy, our templates reduce the risks associated with project management and simplify the implementation process. Say goodbye to the guesswork and benefit from a proven, step-by-step approach to your AI projects. Choose efficiency, reliability, and success with our standard AI project templates.
Crossover of multiple arbitrage targets
Maximize your profits with our AI-driven arbitrage solutions. Our technology monitors multiple markets to identify safe and profitable arbitrage opportunities with accuracy and speed. No longer waste time searching for viable strategies. Our AI-powered approach streamlines the process and gives you a competitive edge in the market. Choose success and growth with our crossover of multiple arbitrage targets.
Special Highlights
Discover the power of our cutting-edge trading solutions. With hundreds of trading papers published every year, it can be difficult to know which methods will truly deliver results. Our special highlight sets us apart from the rest. We bring together rigorous theoretical analysis, standard AI project management templates, and a deep understanding of 100 years of trading history to create a truly reliable and controllable AI-driven trading system. Our automated methods, combined with deep learning, ensure that the results of our backtests can be accurately reproduced in real-world scenarios. With the option for future technology updates, you can trust that you’re always ahead of the curve. Let us bring stability and growth to your trading strategy with our innovative solutions.
Anti-Black-Swan Technique
Say goodbye to unpredictable losses with our Anti-Black-Swan Technique. Black swans events can be a major source of headaches in the trading world, but our innovative solution is designed to tackle them head-on. Using reinforcement learning, our AI system develops its own adversarial capabilities, making it capable of handling even the most unexpected market movements. Backtesting and real-money testing have confirmed the efficacy of our technique. In fact, we welcome black swan events as they often bring substantial profits, regardless of market trends. Trust us to help you navigate even the stormiest waters with confidence.
Standard AI Project Template
Get ahead of the competition with our Standard AI Project Template. After a decade of experience and hundreds of trial and error projects, we have finally succeeded in creating a rigorous mathematical framework for applying AI to the trading industry. Our approach seamlessly blends the best of both worlds – the traditional and proven technical indicators and the latest advancements in AI technology. With our standard project template, you can easily manage your trading projects, streamline your resource consumption, and effectively plan and track your results. Rest assured that you will see foreseeable returns and consistent performance with our solution
Trading-Oriented Neural Network
Upgrade your trading strategy with our Trading-Oriented Neural Network. With over a century of trading knowledge and the latest advancements in AI technology, we have created a solution that seamlessly integrates the best of both worlds. Our approach leverages the conclusions from past trading techniques and enhances them with deep learning, resulting in a system that can improve the accuracy and efficiency of indicator-based trading. With the ability to retain the original trading logic, our solution offers a quick and seamless upgrade to state-of-the-art AI technology.
FAQ
Q 1. who is the creator of technical indicator for trading?
A: Technical indicators are mathematical calculations that are used to analyze financial market data, such as price, volume, and open interest, to identify trends and patterns that may be useful for making trading decisions. The concept of technical analysis and the use of technical indicators for trading can be traced back to the work of Charles Dow, the founder of Dow Jones & Company, in the late 19th century. Dow developed a number of principles for analyzing market trends, and these principles formed the basis for the development of many of the technical indicators that are still in use today.
Other notable figures who have contributed to the development of technical indicators include:
John Bollinger: Bollinger is a financial analyst and the creator of Bollinger Bands, a technical indicator used to measure the volatility of a financial instrument.
George Lane: Lane is credited with the development of the Stochastic Oscillator, a technical indicator used to identify potential trend reversal points.
J. Welles Wilder Jr.: Wilder is the creator of a number of popular technical indicators, including the Average True Range, the Relative Strength Index, and the Parabolic SAR.
Alexander Elder: Elder is a psychiatrist and trading coach who has written extensively on technical analysis and the use of technical indicators in trading. He is the author of several books on the subject, including „Come into My Trading Room“ and „Trading for a Living.“
Q 2. The defintion of AI Quant Trading in a mathematical way?
A: AI quant trading can be defined mathematically as the use of machine learning algorithms to analyze financial data and make trading decisions based on the results of this analysis. These algorithms may be based on a variety of mathematical techniques, including statistical analysis, optimization, and probability theory.
One common approach to AI quant trading is to use statistical techniques to identify trends and patterns in financial data. For example, a trader might use regression analysis to identify the relationship between different financial indicators and the price of a particular asset. Another approach might be to use clustering algorithms to group similar assets together based on their characteristics.
Other AI quant trading techniques may be based on optimization algorithms, which seek to maximize or minimize some objective function. For example, a trader might use a linear programming algorithm to optimize a portfolio of assets based on the expected return and risk.
Probability theory is also a key component of many AI quant trading strategies. For example, a trader might use a Monte Carlo simulation to estimate the likelihood of different outcomes based on the historical performance of an asset.
Overall, the goal of AI quant trading is to use advanced mathematical techniques to identify trading opportunities and make informed decisions based on the analysis of financial data.
Q 3. How to appy AI to Trading Industry?
A: Artificial Intelligence (AI) has the potential to greatly enhance and optimize the process of trading. To apply AI into trading, it’s important to first have a solid understanding of the market and the financial data that is relevant to making informed trading decisions. This information can then be fed into AI algorithms, such as machine learning models, to help identify patterns and make predictions about future market trends.
Once the data has been gathered, the next step is to train the AI algorithm. This involves feeding the algorithm a large amount of historical data and training it to recognize patterns and make predictions. The AI algorithm can then be tested on new data to evaluate its accuracy and refine it as needed.
Another important factor to consider when applying AI to trading is the development of an appropriate trading strategy. This could involve incorporating technical indicators, such as moving averages and Bollinger Bands, into the AI algorithm to help make informed trading decisions. It’s also important to ensure that the AI algorithm is integrated with the existing trading system and can communicate with the necessary trading platforms and APIs.
Finally, it’s important to continually monitor and evaluate the performance of the AI algorithm. This can be done by tracking its trading performance over time and making necessary adjustments to the algorithm to optimize its performance.
In conclusion, applying AI to trading requires a combination of technical expertise in AI and financial markets, as well as an understanding of the market and the data that is relevant to making informed trading decisions. It’s a process that involves careful planning, development, testing, and ongoing refinement to ensure that the AI algorithm is able to consistently make informed trading decisions that lead to profitability.
Q 4. What’s the pain point of using AI in trading?
A: pain point of using AI in trading Using Artificial Intelligence (AI) in trading can be challenging and come with several pain points. One of the biggest challenges is ensuring the accuracy and reliability of the AI system. There is a need to have a thorough understanding of the financial market and the data being used to train the AI system. If the data used is not representative or outdated, it can lead to poor performance of the AI system and cause significant losses.
Another pain point of using AI in trading is the need for high-quality data pre-processing and feature engineering. The AI system needs to be fed the right kind of data in the right format to be able to make accurate predictions and decisions. This requires a good understanding of the data, the market and the business problem being solved.
The complexity of the AI system can also pose a challenge. AI systems can become complex and difficult to maintain, requiring specialized knowledge and resources. Additionally, the deployment of the AI system can also be challenging, as it requires a good understanding of the technology and the financial market.
Finally, the use of AI in trading also raises ethical and regulatory concerns, as it can impact the financial market and its participants. As AI technology continues to evolve, it is important to have proper oversight and regulation in place to ensure its safe and responsible use.
Q 5. How to make sure that the AI bot will produce profit continuously?
A: Ensuring that an AI bot will produce profits continuously is a complex task that requires a thorough understanding of the AI technology and the market environment. Some key factors that contribute to the success of an AI trading system include:
- Data quality and quantity: The AI model needs to be trained on high-quality and relevant data, which includes market data and historical financial data, to make accurate predictions and decisions.
- Model selection and design: The AI model used for trading should be carefully selected and designed to suit the specific requirements of the trading system, taking into account factors such as market volatility, transaction costs, and other constraints.
- Risk management: An effective risk management strategy should be in place to ensure that the AI system is not exposed to excessive risk, especially during periods of market volatility. This may involve setting stop-loss orders, adjusting position sizes, or diversifying the portfolio.
- Backtesting and evaluation: Before deploying an AI trading system, it is important to backtest the model to evaluate its performance and make any necessary adjustments. This can involve running simulations of the AI model on historical data to determine its accuracy and profitability.
- Continual monitoring and adjustment: The AI system should be monitored regularly to ensure that it is performing as expected and making the right decisions. Any necessary adjustments should be made in a timely manner to ensure that the system remains profitable and efficient.
In summary, applying AI to trading is a complex task that requires a multi-faceted approach that covers data, model design, risk management, evaluation, and monitoring. It is essential to take the time to understand the market, the technology, and the specific requirements of the trading system, to ensure that the AI trading system is profitable and sustainable in the long term.
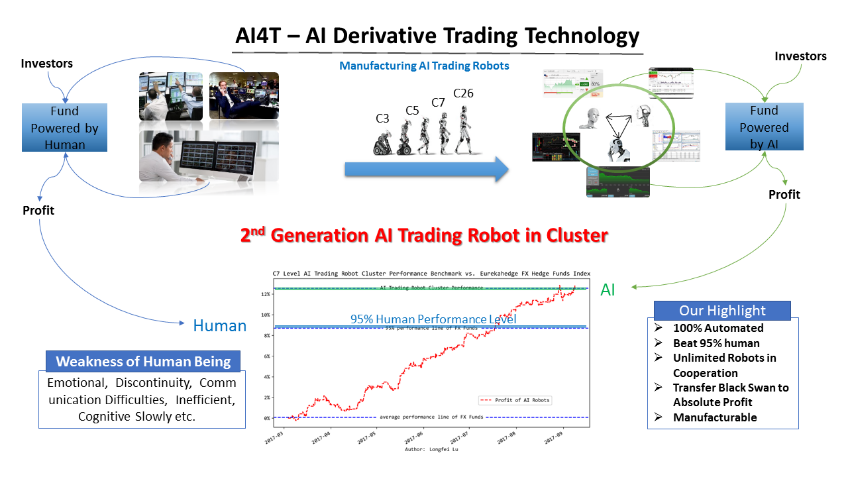
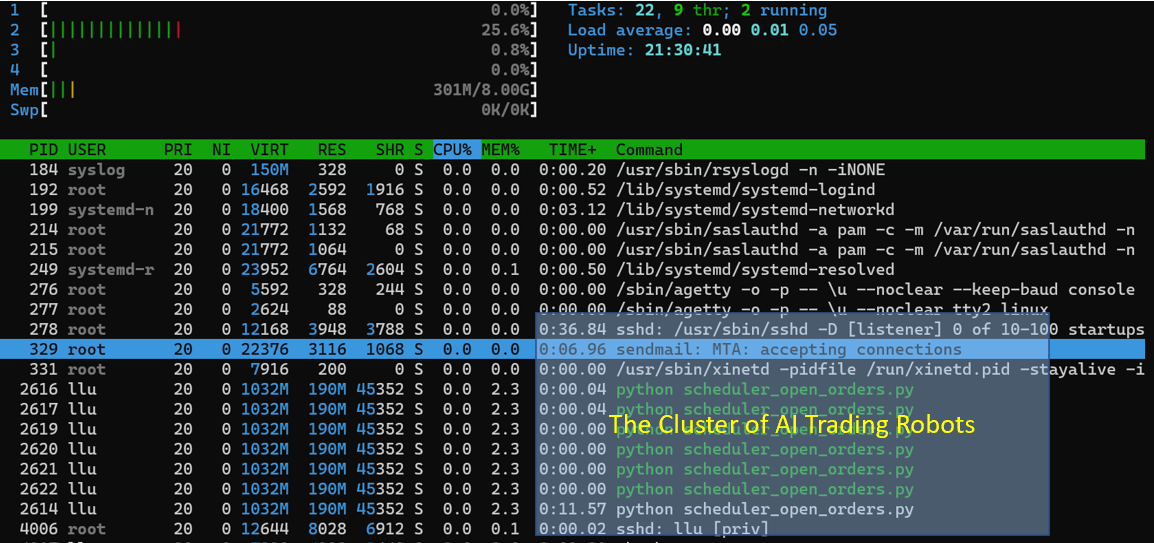
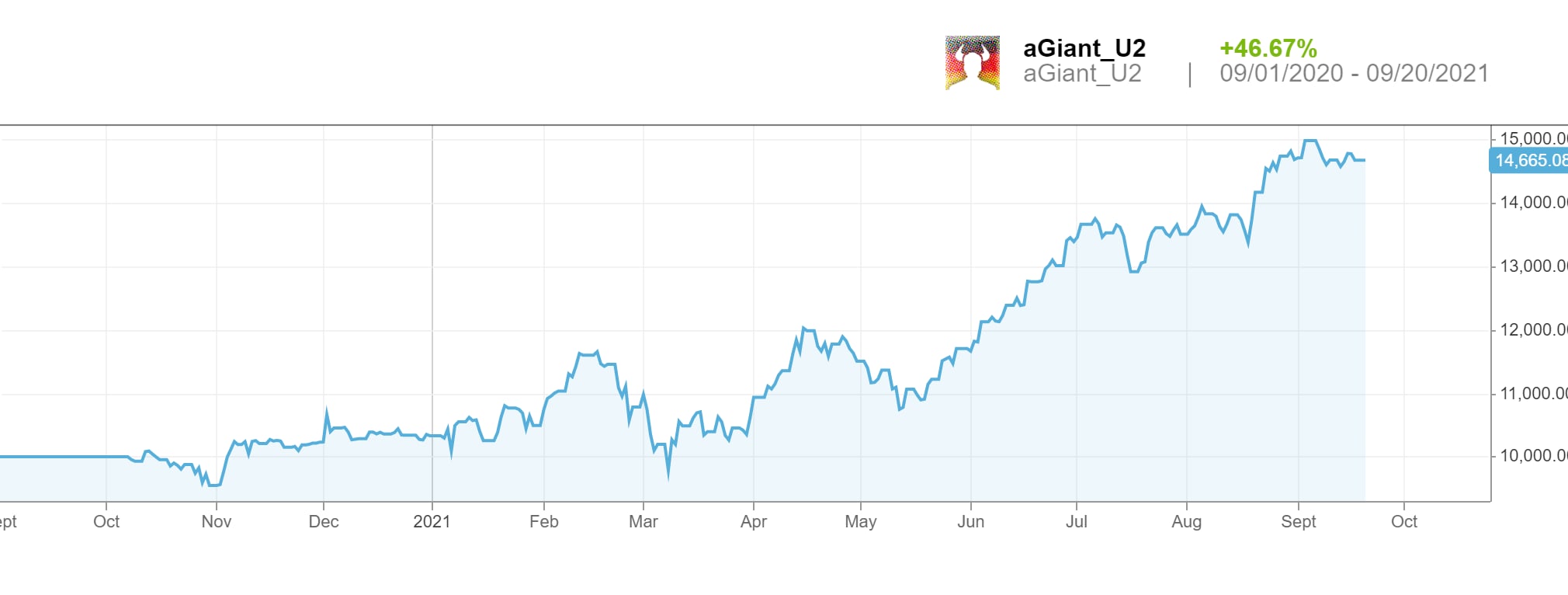
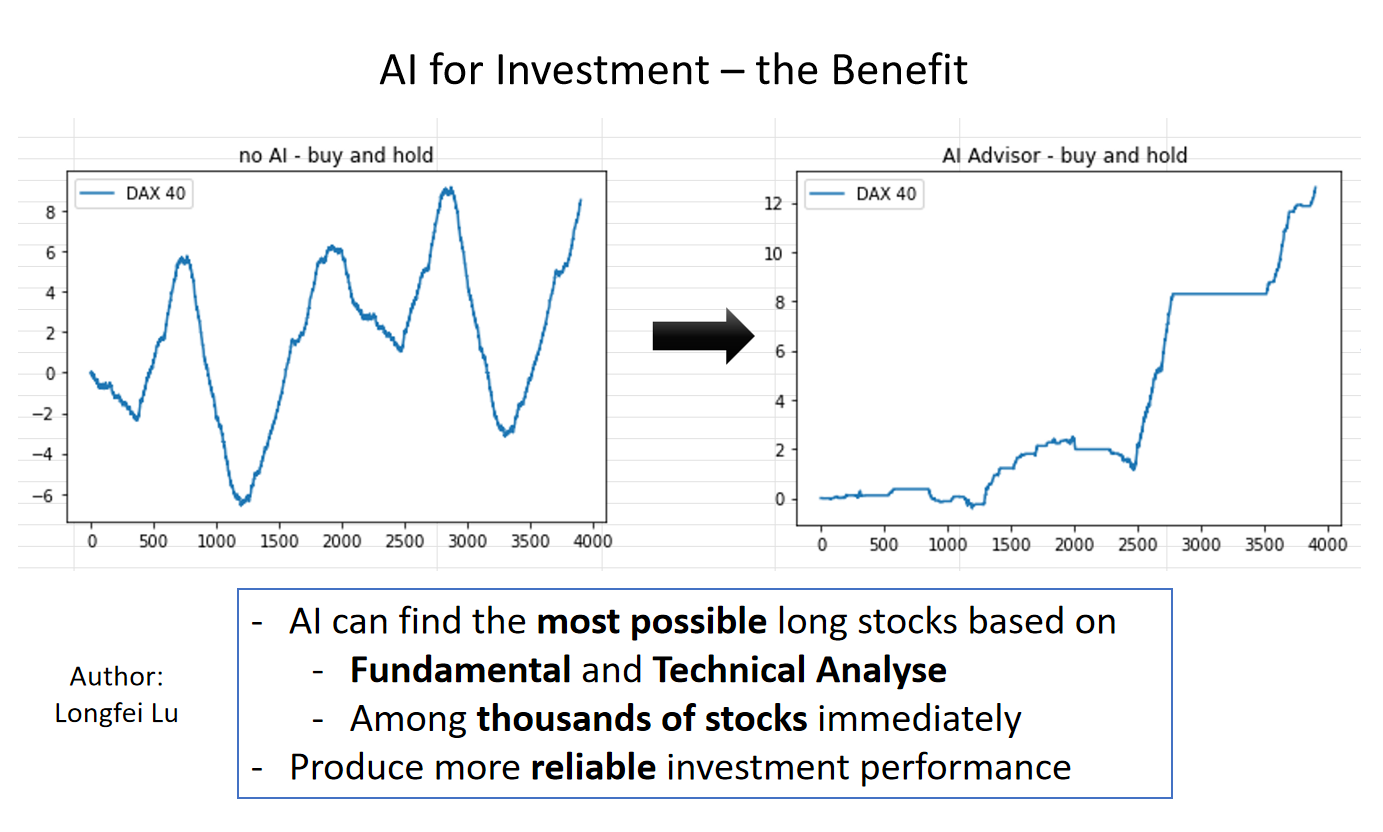
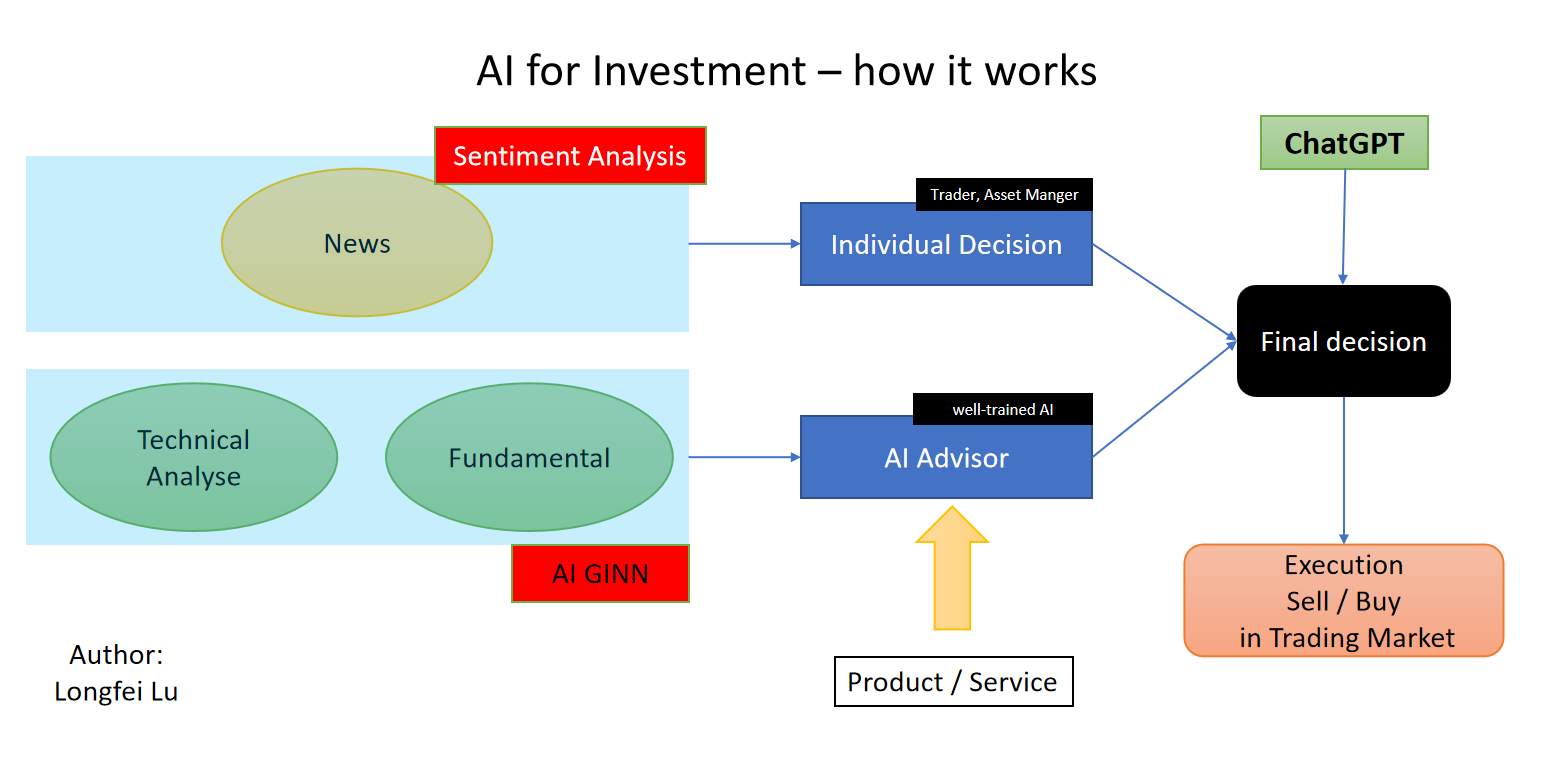